Overview
This five-day course is designed for those who want to broaden their mathematical knowledge beyond Statistics and Probability for Data Science. Perhaps with the aim of increasing your understanding of existing Python or R packages, or in order to progress towards developing your own methods and algorithms.
This course introduces concepts with practical programming exercises and covers essential topics for Data Science such as Vectors, Matrices, Calculus, and how these are applied in common Data Science problems for business uses.
You will also have the opportunity to learn a wide range of notations and terminology in order to support you in reading books and journal articles intended for Data Science audiences.
Following this, you will be exposed to topics to introduce you to areas at the cutting edge of Data Science: Graph analytics, AI and Deep Learning, and Quantum Computing Development.
For those wishing to certify as Data Scientists this course is aligned with many certifications and professional frameworks in order to support you on your learning journey.
It is expected that you will have experience with Python or R and Statistics for Data Analysis. It would be beneficial, but not essential to be familiar with algebra and functions such as quadratics and trigonometry.
Target Audience
This course is for individuals who have experience with Python or R in the context of a Data Science or related field.
- Data Scientists
- Software Developers
- Advanced Data Analysts
- ML / AI Data Engineers
Prerequisites
Delegates must be existing Python or R Data users who have attended:
- Python or R for Data Handling (QADHPYTHON or QADHR) and
- Statistics for Data Analysis with Python or R (QASDAPY or QASDAR)
- or have a similar level of knowledge.
Outline
1. Introduction to Mathematics for Data Science
- Discuss the source code in commonly used Data Science Python or R libraries
- Identify use cases for a range of Mathematical techniques used in Data Science, Machine Learning, Big Data, AI, and Quantum Computing such as Probability and Statistics, Linear Algebra (Vectors and Matrices), Calculus, Graphs and Networks, and Complex Numbers.
2. Matrices and Programming
- Describe the structure of a Matrix.
- Use Python NumPy Arrays or R Matrices to build Mathematically structured Matrices
- Explore NumPy or R functions to create various Matrices
3. Matrix Arithmetic
- Explain the conditions that matrices need to meet in order to apply mathematical operations.
- Apply Arithmetic with matrices using NumPy or R
- Apply Multiplication with scalars and matrices using NumPy or R
4. Inverses and Solving Simultaneous Equations
- Apply a Multiplicative Inverse for dividing matrices
- Calculate the determinant of a matrix
- Solve Simultaneous equations using matrices
- Interpret the meaning of a zero determinant in the context of solving simultaneous equations
5. Matrix Transformations
- Identify how matrices can be transformed
- Perform multiple vector transformations using NumPy or R
- Calculate and interpret eigenvalues and eigenvectors
6. Vectors and Dot Product
- Identify the purpose of vectors and dot products in Data Science
- Create and visualise vectors using NumPy or R
- Calculate dot products and use visualisations to explain how dot product can be used to indicate how similar two columns or rows of data are
7. Vector Spaces
- Describe what a vector basis is
- Identify possible vectors that can be used to form a basis for a particular space and choose the most efficient basis
- Use dot product to check the vectors in the basis are orthogonal
8. Differentiation and Gradients
- Identify notation used for differentiation
- Describe how gradients can be calculated numerically (using NumPy or R) or algebraically
- Discuss stationary points and the second differential
- Recognise the Chain rule and Partial Differentiation
9. Gradient Descent
- Identify types of stationary points
- Describe the concept behind a gradient descent algorithm
- Use differentiation and NumPy or R to locate a minimum point using a gradient descent algorithm
10. Linear Regression with Gradient Descent
- Calculate residuals and identify functions that could be used to aggregate these to select a loss function for performing linear regression
- Use gradient descent to calculate a regression line
11. Set Building and Mathematical Notation
- Identify a wide range of mathematical notation and its purpose
- Create sets using set builder notation
- Identify symbols used for common functions and processes
- Practice interpreting formulae or expressions in mathematical journal articles or books
12. Graphs, Networks, Deep Learning and Neural Network Calculations
- Investigate software packages for Deep Learning
- Identify parts of Graphs and Networks using Mathematical Terminology
- Investigate how Graphs and Networks are used to provide an abstraction to a problem in order to interpret algorithms including Big Data Analysis problems
- Examine how Matrices and Calculus are used to carry out Neural Network Calculations
13. Complex Numbers and Quantum Computing Development
- Store complex numbers in either Python or R
- Explain the meaning of i, complex numbers, complex conjugates, and perform arithmetic calculations with complex numbers by checking results using Python or R
- Match quantum computing terms to their meanings. Including qubit, state vector, Hilbert space, superposition, quantum gate, measurement, quantum circuit, unitary transformation
- Represent quantum gates as matrices and calculate the effect of transforming basis states.
Related learning
Data Science Learning Pathways can be selected by choosing either Python or R and a Cloud Platform certification:
- QAIDSDP Introduction to Data Science for Data Professionals
- Sourcing and handling data:
- QADHPYTHON Data Handling with Python
- QADHR Data Handling with R
- QAPDHAI Python Data Handling with AI APIs
- Statistics for Data Analysis:
- QASDAPY Statistics for Data Analysis with Python
- QASDAR Statistics for Data Analysis with R
- Programming and Software Development skills:
- QAPYTH3 Python Programming
- QARPROG R Programming
- Machine Learning Development:
- QADSMLP Data Science and Machine Learning with Python
- QADSMLR Data Science and Machine Learning with R
- Forecasting:
- QATSFP Time Series and Forecasting with Python
- QATSFR Time Series and Forecasting with R
Suggested courses leading to Certification:
- MDP100 Designing and Implementing a Data Science Solution on Azure (DP-100)
- AMWSMLP Machine Learning Pipelines on AWS
- GCPMLGC Machine Learning on Google Cloud
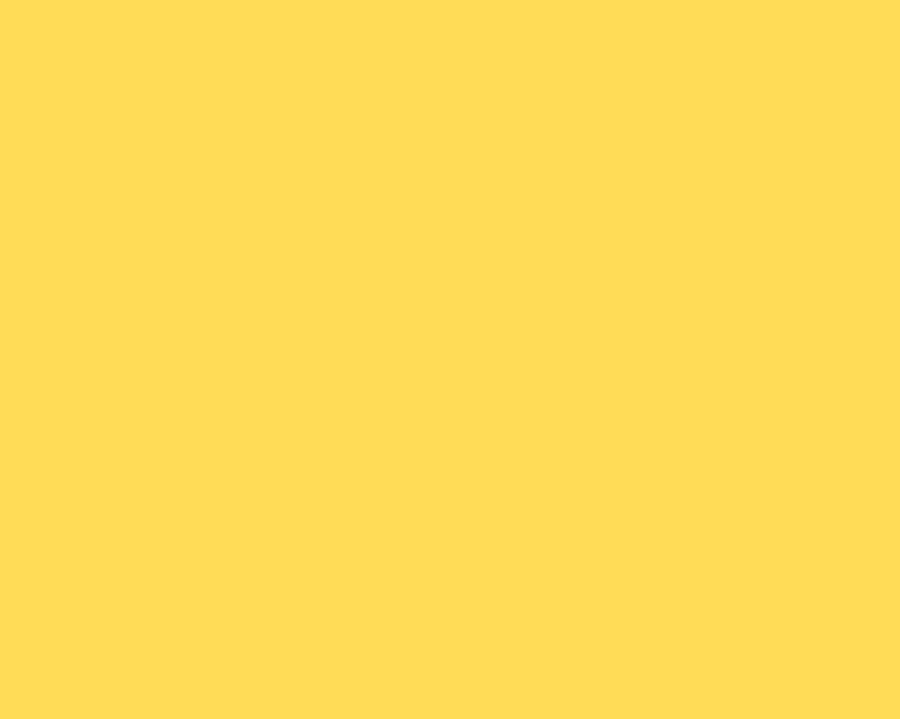
Frequently asked questions
How can I create an account on myQA.com?
There are a number of ways to create an account. If you are a self-funder, simply select the "Create account" option on the login page.
If you have been booked onto a course by your company, you will receive a confirmation email. From this email, select "Sign into myQA" and you will be taken to the "Create account" page. Complete all of the details and select "Create account".
If you have the booking number you can also go here and select the "I have a booking number" option. Enter the booking reference and your surname. If the details match, you will be taken to the "Create account" page from where you can enter your details and confirm your account.
Find more answers to frequently asked questions in our FAQs: Bookings & Cancellations page.
How do QA’s virtual classroom courses work?
Our virtual classroom courses allow you to access award-winning classroom training, without leaving your home or office. Our learning professionals are specially trained on how to interact with remote attendees and our remote labs ensure all participants can take part in hands-on exercises wherever they are.
We use the WebEx video conferencing platform by Cisco. Before you book, check that you meet the WebEx system requirements and run a test meeting to ensure the software is compatible with your firewall settings. If it doesn’t work, try adjusting your settings or contact your IT department about permitting the website.
How do QA’s online courses work?
QA online courses, also commonly known as distance learning courses or elearning courses, take the form of interactive software designed for individual learning, but you will also have access to full support from our subject-matter experts for the duration of your course. When you book a QA online learning course you will receive immediate access to it through our e-learning platform and you can start to learn straight away, from any compatible device. Access to the online learning platform is valid for one year from the booking date.
All courses are built around case studies and presented in an engaging format, which includes storytelling elements, video, audio and humour. Every case study is supported by sample documents and a collection of Knowledge Nuggets that provide more in-depth detail on the wider processes.
When will I receive my joining instructions?
Joining instructions for QA courses are sent two weeks prior to the course start date, or immediately if the booking is confirmed within this timeframe. For course bookings made via QA but delivered by a third-party supplier, joining instructions are sent to attendees prior to the training course, but timescales vary depending on each supplier’s terms. Read more FAQs.
When will I receive my certificate?
Certificates of Achievement are issued at the end the course, either as a hard copy or via email. Read more here.